Impact of Low-Rank Adaptation on Backdoor Attacks in a Federated Learning Context
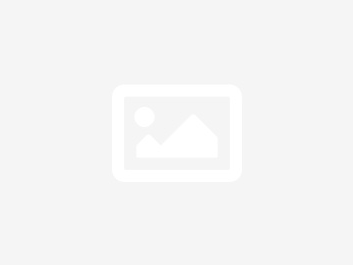
Description
Federated learning (FL) has emerged as a promising paradigm for collaborative model training while improving the privacy of local data. However, the decentralized nature of FL introduces significant vulnerabilities to backdoor attacks, where malicious participants can compromise the global model's integrity. A major trend is the use of federated learning to adapt (fine-tuning) large-scale models (e.g., visual transformer, llm) to more focused tasks. Such adaptation processes usually rely on parameter-efficient fine-tuning (PEFT) mechanisms in order to drastically reduce the complexity of the task. A state-of-the-art approach is the well-known Low-Rank Adapatation (LoRA). Here, we propose the first study investigating the impact of LoRA on backdoor attacks, that are powerful targeted data poisoning threats. Although LoRA seems to constrain standard backdoor attacks and significantly reduces their persistence, we show that LoRA provides a false sense of robustness since more advanced, adaptative attacks can thwart this phenomenon. We propose several experiments and analysis using Visual Transformer models and state-of-the-art FL-oriented backdoor attacks.